Generative AI (GenAI) is a revolutionary technology with the potential to transform various industries. However, to leverage its full potential, enterprises need to adapt their data strategies. This blog post explores the challenges and opportunities at the intersection of enterprise data strategy and GenAI, providing a roadmap for successful integration.
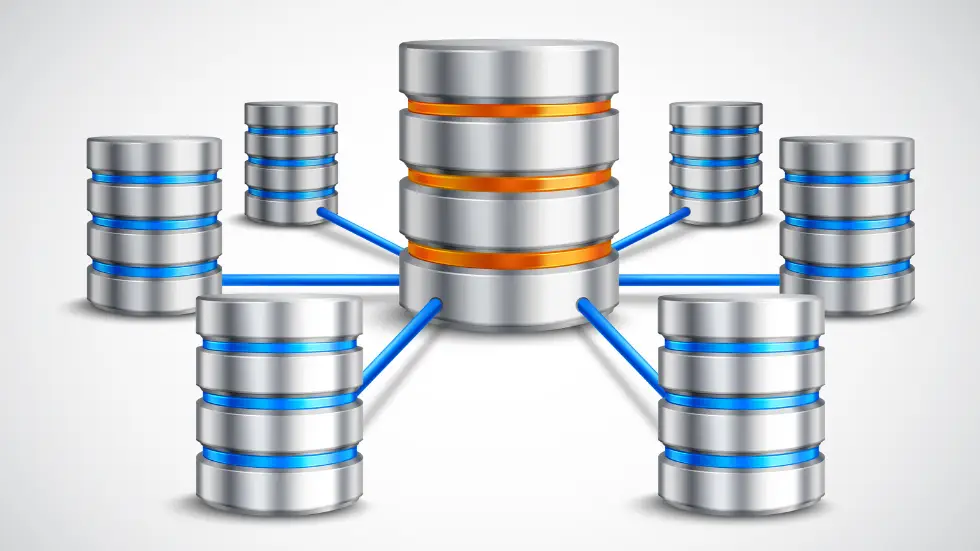
The Challenge: Data Silos Hinder Generative AI for Enterprise Data Strategy
Generative AI thrives on large amounts of high-quality data. Traditional data strategies often struggle due to:
- Data Silos and Accessibility: Fragmented data across disparate systems hinders access and integration for GenAI models.
- Data Quality and Consistency: Inconsistent or biased data can lead to unreliable and subpar GenAI outputs.
- Data Security and Privacy: Balancing data access with robust security and privacy measures is crucial for responsible AI development.
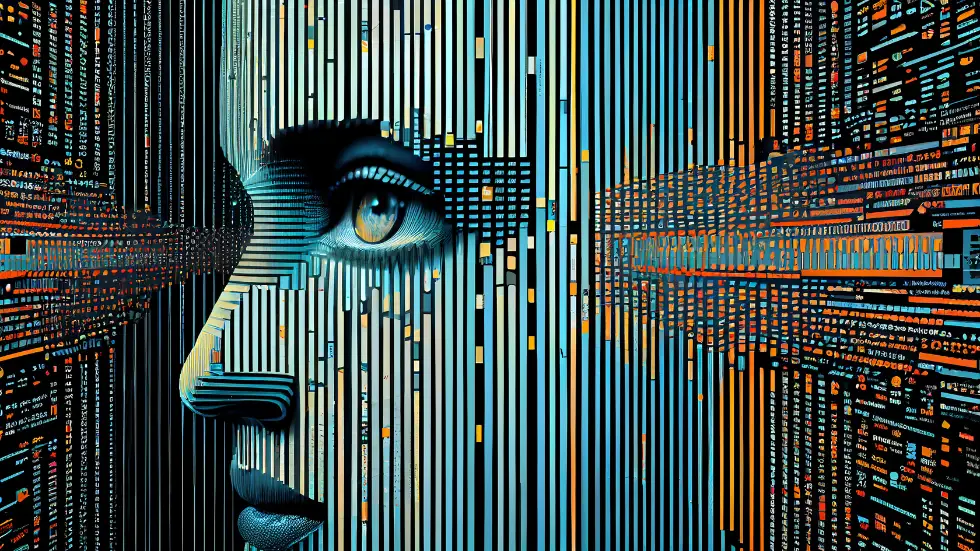
The Opportunity: A Data-Centric Approach for Generative AI
To unlock GenAI’s potential, enterprises must embrace a data-centric approach:
- Data Democratization: Break down data silos and create a unified data platform for seamless GenAI model access and integration.
- Data Governance and Quality Management: Implement data governance frameworks to ensure data quality, consistency, and compliance.
- Data Labeling and Annotation: Invest in data annotation to enrich existing data with relevant labels and context for supervised learning algorithms in GenAI.
- Synthetic Data Generation: Utilize synthetic data generation to supplement real-world data and address privacy concerns.
- Continuous Learning and Feedback Loops: Establish mechanisms for continuous learning and feedback to improve GenAI model performance and adaptation.
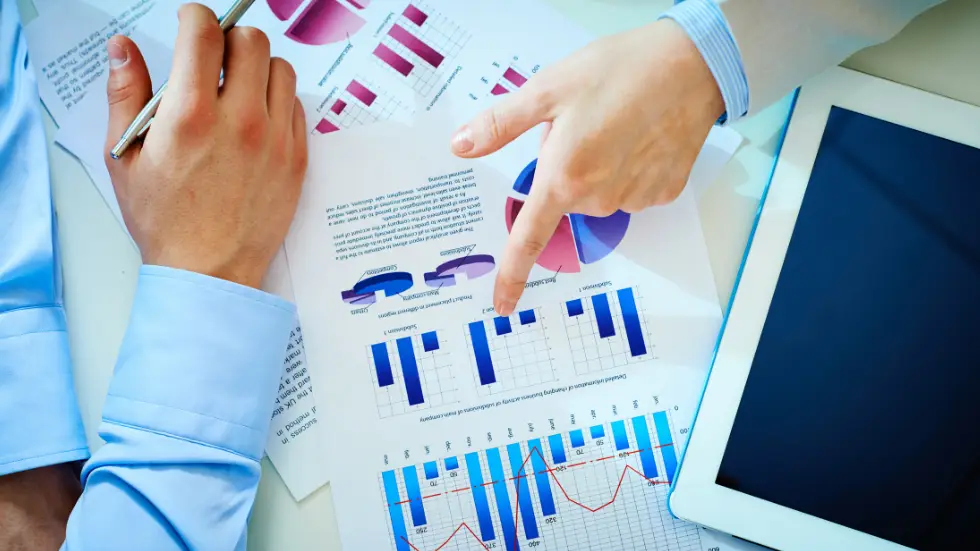
Specific Use Cases and Data Strategy Adaptations
Data strategy adaptations will vary depending on the GenAI use case:
- Product Development: Design data, user feedback data, and historical sales data are crucial. Focus on data integration and quality management.
- Marketing and Sales: Customer data, market research data, and campaign performance data are necessary. Prioritize data governance and privacy-preserving techniques.
- Research and Development: Access to scientific datasets, research papers, and experimental data is essential. Focus on data security and collaboration frameworks.
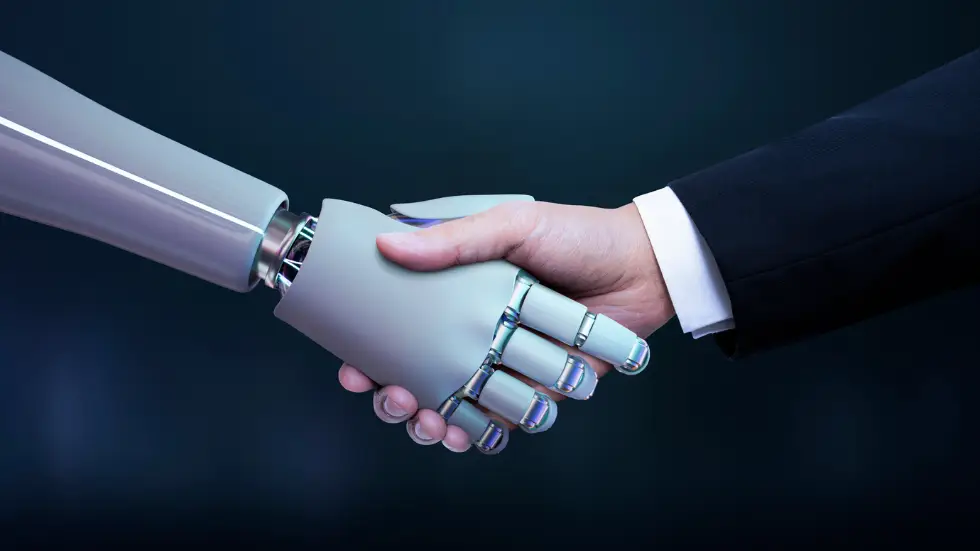
The Road Ahead: Collaboration for Successful Generative AI Integration
The successful integration of GenAI requires collaboration between:
- Data Scientists and IT Teams: Collaborate to harmonize data infrastructure for seamless data access and GenAI model integration.
- Business Leaders and Domain Experts: Provide context and guidance on data labeling, annotation, and feedback loops for relevant and effective GenAI outputs.
- Regulatory Bodies and Legislators: Develop and implement ethical frameworks and legal guidelines for responsible AI development and data usage in the GenAI landscape.
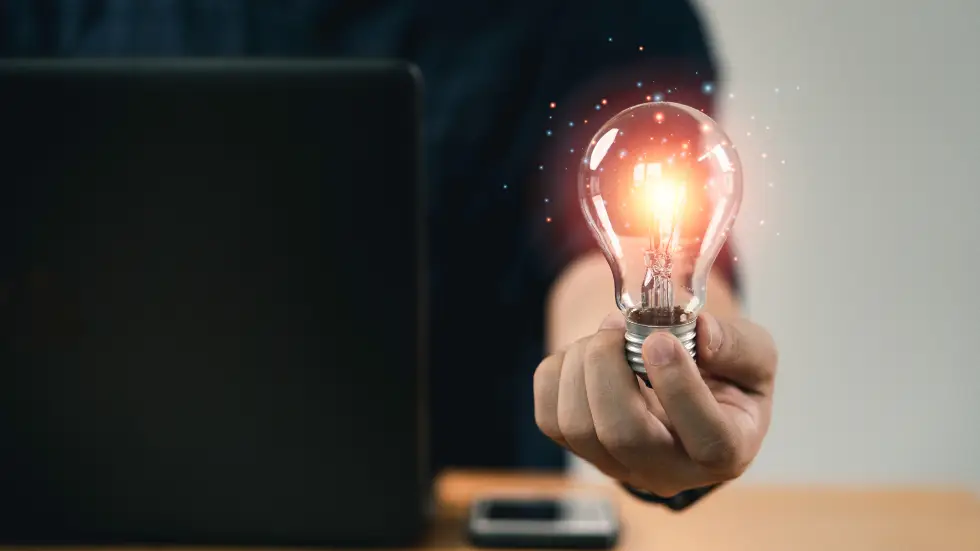
Conclusion
By embracing a data-centric approach, investing in data infrastructure and governance, and fostering collaboration, enterprises can unlock the immense potential of GenAI and propel themselves towards a future driven by innovation and intelligent automation. Remember, the journey towards successful GenAI integration is ongoing, requiring continuous learning, adaptation, and a commitment to responsible AI development practices.
Additional Resources: